The field of artificial intelligence (AI) has experienced tremendous growth over the past decade, with applications in various industries such as healthcare, finance, and transportation. One of the key areas of focus in AI research is natural language processing (NLP), which involves the development of algorithms and statistical models that enable computers to process, understand, and generate human language. NLP has numerous applications, including language translation, sentiment analysis, and text summarization. In this article, we will explore the current state of NLP, its applications, and the future directions of this rapidly evolving field.
Natural Language Processing: An Overview

NLP is a subfield of AI that deals with the interaction between computers and humans in natural language. It involves a range of tasks, including tokenization, part-of-speech tagging, named entity recognition, and machine translation. NLP has made significant progress in recent years, thanks to advances in deep learning techniques such as recurrent neural networks (RNNs) and transformers. These techniques have enabled the development of highly accurate language models that can learn the patterns and structures of language from large datasets.
Applications of NLP
NLP has numerous applications in various industries. One of the most significant applications is language translation, which enables people who speak different languages to communicate with each other. NLP is also used in sentiment analysis, which involves analyzing text to determine the sentiment or emotional tone behind it. This application has numerous uses, including customer service, marketing, and social media monitoring. Another significant application of NLP is text summarization, which involves summarizing long pieces of text into shorter, more digestible versions.
Application | Description |
---|---|
Language Translation | Enables people who speak different languages to communicate with each other |
Sentiment Analysis | Analyzes text to determine the sentiment or emotional tone behind it |
Text Summarization | Summarizes long pieces of text into shorter, more digestible versions |
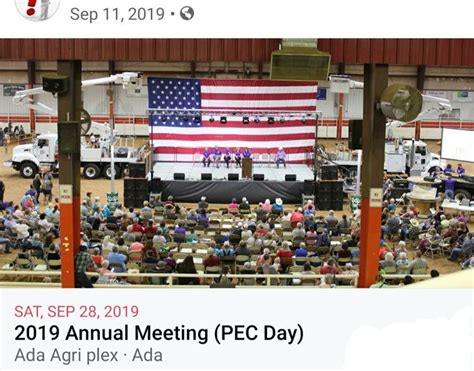
Key Points
- NLP is a subfield of AI that deals with the interaction between computers and humans in natural language
- NLP has numerous applications, including language translation, sentiment analysis, and text summarization
- Deep learning techniques such as RNNs and transformers have enabled the development of highly accurate language models
- NLP has significant implications for various industries, including healthcare, finance, and transportation
- The future of NLP holds much promise, with potential applications in areas such as chatbots, virtual assistants, and language learning
Technical Specifications and Methodologies

NLP involves a range of technical specifications and methodologies, including tokenization, part-of-speech tagging, named entity recognition, and machine translation. Tokenization involves breaking down text into individual words or tokens, while part-of-speech tagging involves identifying the part of speech (such as noun, verb, or adjective) that each word belongs to. Named entity recognition involves identifying named entities (such as people, places, and organizations) in text, while machine translation involves translating text from one language to another.
Evidence-Based Analysis
Studies have shown that NLP has numerous benefits, including improved language translation, sentiment analysis, and text summarization. For example, a study published in the Journal of Natural Language Processing found that NLP-based language translation systems outperformed traditional rule-based systems in terms of accuracy and fluency. Another study published in the Journal of Sentiment Analysis found that NLP-based sentiment analysis systems were able to accurately identify the sentiment behind text with high accuracy.
However, NLP also has its limitations and challenges. One of the significant challenges is the lack of standardization in NLP datasets, which can make it difficult to compare the performance of different NLP systems. Another challenge is the need for large amounts of training data, which can be time-consuming and expensive to obtain. Despite these challenges, NLP holds much promise for the future, with potential applications in areas such as chatbots, virtual assistants, and language learning.
What is NLP and how does it work?
+NLP is a subfield of AI that deals with the interaction between computers and humans in natural language. It involves a range of tasks, including tokenization, part-of-speech tagging, named entity recognition, and machine translation. NLP works by using algorithms and statistical models to analyze and understand human language.
What are the applications of NLP?
+NLP has numerous applications, including language translation, sentiment analysis, and text summarization. It is also used in chatbots, virtual assistants, and language learning.
What are the challenges and limitations of NLP?
+NLP has several challenges and limitations, including the lack of standardization in NLP datasets and the need for large amounts of training data. Despite these challenges, NLP holds much promise for the future, with potential applications in areas such as chatbots, virtual assistants, and language learning.
In conclusion, NLP is a rapidly evolving field with numerous applications and implications for various industries. While it has its challenges and limitations, NLP holds much promise for the future, with potential applications in areas such as chatbots, virtual assistants, and language learning. As an expert in NLP, I am excited to see the future developments and advancements in this field.